With the advent of large language models, machine translation (MT) has become a widely used, but little understood, tool for accessing historical and multilingual texts. While models like GPT, Claude, and Deepseek increasingly enable translation of low-resource and ancient languages, critical questions remain about their evaluation, optimal model selection, and the value of domain-specific training and retrieval-augmented generation setups. While AI models like GPT, Claude, and Deepseek are improving translation capabilities for low-resource and ancient languages, researchers still face important questions about how to evaluate their performance, which models work best, and whether specialized training approaches provide meaningful improvements in translation quality. This study introduces a comprehensive evaluation dataset for Buddhist Chinese to English translation, comprising 2,662 bilingual data points from 32 texts that have been selected to represent the full breadth of the Chinese Buddhist canon. We evaluate various computational metrics of translation quality (BLEU, chrF, BLEURT, GEMBA) against expert annotations from five domain specialists who rated 182 machinegenerated translations. Our analysis reveals that LLM-based GEMBA scoring shows the strongest correlation with human judgment, significantly outperforming traditional metrics. We then benchmark commercial models (GPT-4 Turbo, Claude 3.5, Gemini), opensource models (Gemma 2, Deepseek-r1), and a domain-specialized model (Gemma 2 Mitra) using GEMBA. Our results demonstrate that domain-specific training enables openweights models to achieve competitive performance with commercial systems, while also showing that retrieval-augmented generation (RAG) significantly improves translation quality for the best performing commercial models.
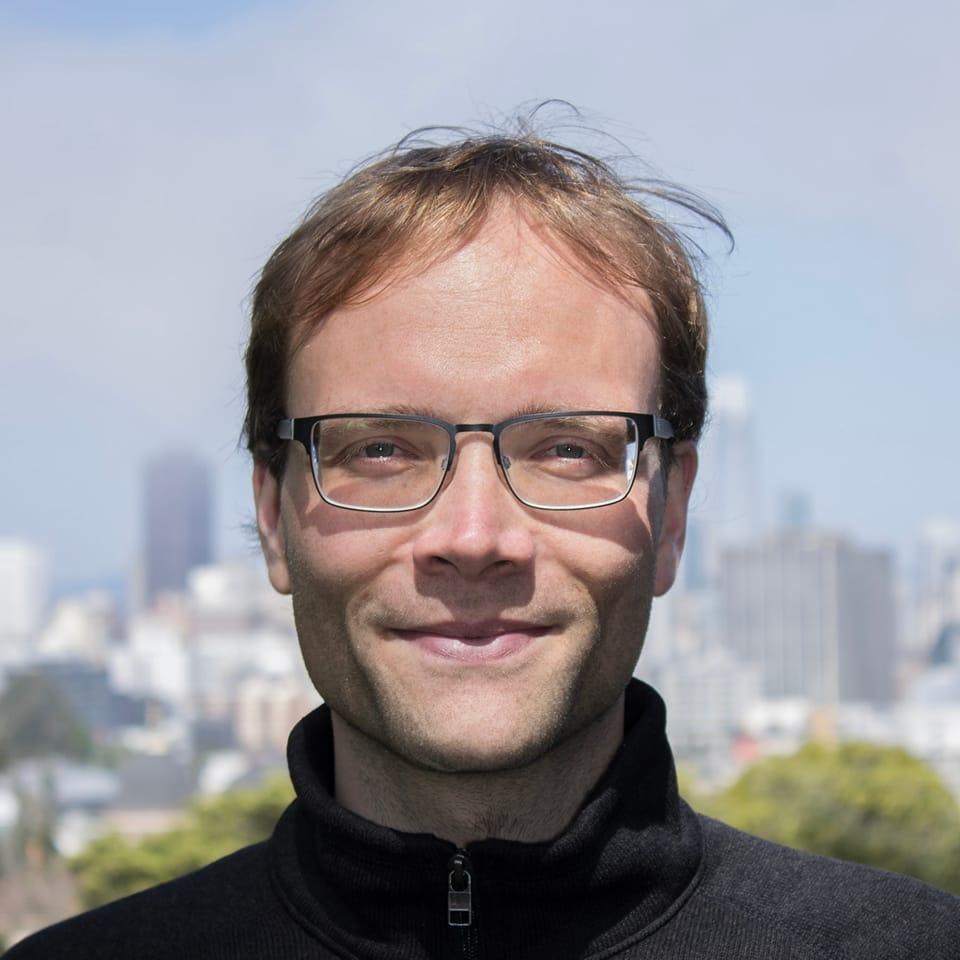
Sebastian Nehrdich
Research @ BAIR, UC Berkeley; PhD candidate @ University of Duesseldorf
- Berkeley, CA
- UC Berkeley
- Google Scholar
- Github
- X (formerly Twitter)